Qiang Zhang's Website
Research & Initiatives
I'm Qiang Zhang, and my current research interests include biological signal-based neuromusculoskeletal modeling, human motion intent detection,
Lyapunov-based nonlinear control/adaptive control, machine learning-based control, surface electromyography/ultrasound imaging processing, and their applications to wearable robotic devices’ control and next-generation healthcare.
I envision building on my research efforts on biological signal-inspired control/estimation theory and application to assistive, rehabilitative, and industrial robotic devices, human-robot interaction, multi-mode human motion intent or muscle strength prediction, and machine learning-based automatic feature tracking from ultrasound imaging technologies.
Reinforcement learning-based robotic assistance personalization control
Powered exoskeletons are promising devices to improve the walking patterns of people with neurological impairments. Providing personalized external assistance for human locomotion though is challenging due to uncertainties and the time-varying nature of human-robot interaction. To address the robotic assistance personalization control, we have formulated an adaptive optimal control solution based on reinforcement learning for a wearable bilateral hip exoskeleton to automatically tailor control parameters for each individual wearer, such as assistance torque shape profile parameters or impedance parameters, with the goal to minimize the defined cost function of the human-robot symbiotic system. We have also conducted treadmill walking experiments to validate the performance of the proposed control framework on both abled-bodied participants and individuals with paretic stroke. The assistance for both hip extension and flexion during human locomotion has been customized through learning procedures. In addition, we have analyzed the personalized assistance on the hip joint kinematics, kinetics, gait patterns, and muscle activation levels of the symbiotic system.
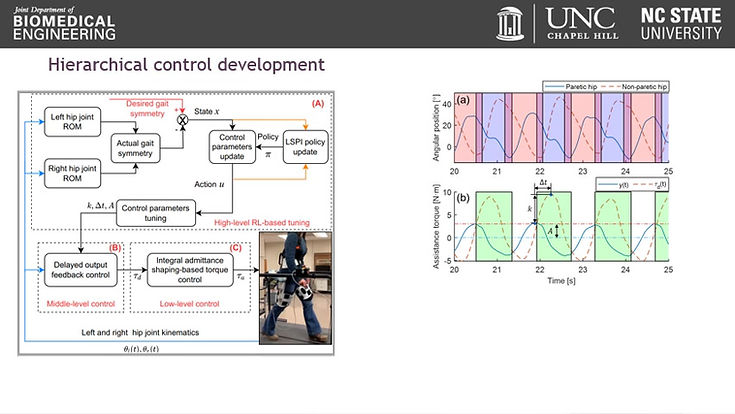
Wearable hip exoskeleton development and torque trajectory tracking
We develop a lightweight wearable bilateral hip exoskeleton with up to four degrees of freedom (DOFs), where the actuation unit for each DOF follows a modular design concept. The embedded load cell for each DOF facilitates
the low-level controller to ensure good torque trajectory tracking control. The fundamental concept for robotic exoskeletons providing assistance depends on reliable and accurate low-level torque trajectory tracking, which is usually challenging during human dynamic locomotion. To address this challenge, we have developed an integral admittance shaping algorithm for the desired torque profile tracking control of a wearable bilateral hip exoskeleton, which could effectively ensure stability and robustness of the output torque control even with unknown external disturbance.
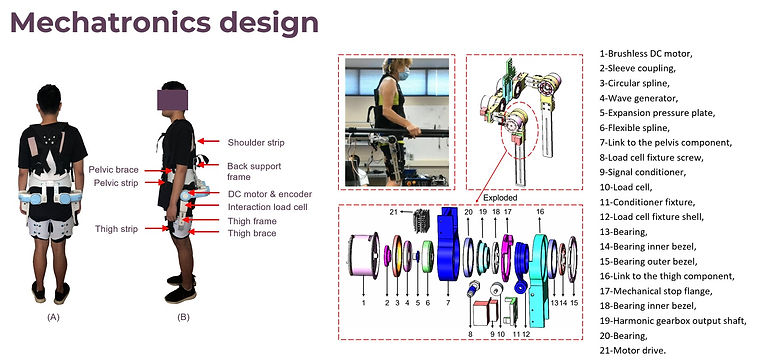
Neuromuscular model-based and model-free human ankle joint motion intent or muscle strength prediction
Traditional human joint motion intent prediction is mainly based on either mechanically intrinsic sensors, like force or torque sensor or inertia movement unit, but usually comes with the drawback of inevitable lagging when compared to the signals from the central nervous system. To address this lagging challenge, surface electromyography (sEMG) signals from peripheral skeletal muscles have been widely applied for human motion intent or muscle strength prediction. However, sEMG has some shortcomings, like cross-talking, interference from adjacent muscles, and the inability to measure deeply located muscles. As an alternative neuromuscular signal, ultrasound (US) imaging has also attracted much attention given the advantage of direct visualization of both superficial and deep muscles. Therefore, we have proposed a dual-mode surrogate that combines both sEMG and US imaging signals in predicting human joint motion intent or muscle strength based on a neuromuscular modeling approach and machine learning approach, including isometric ankle dorsiflexion moment prediction and dynamic net ankle plantarflexion moment prediction during treadmill walking at multiple speeds.
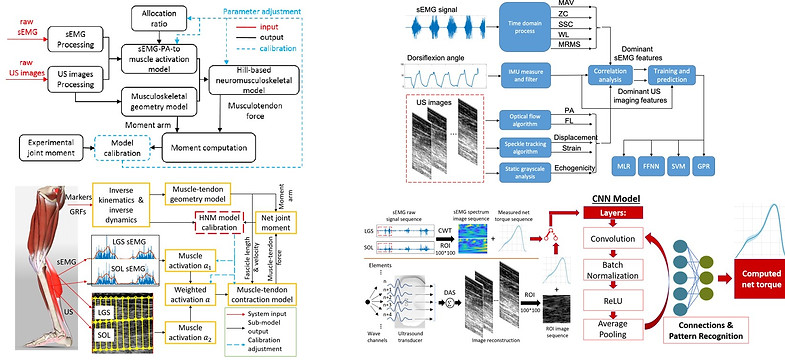
Ultrasound imaging-based control of functional electrical stimulation for Drop Foot Correction
Open-loop or closed-loop functional electrical stimulation has been widely investigated to treat drop foot syndrome, which is typically caused by weakness or paralysis of ankle dorsiflexion. However, conventional closed-loop FES control mainly uses kinematic feedback, which does not directly capture time-varying changes in muscle activation. To address this issue, we have explored the use of US echogenicity as an indicator of FES-evoked muscle activation and hypothesized that including US-derived muscle activation, in addition to kinematic feedback, would improve the closed-loop FES control performance compared to the closed-loop control that relies only on the kinematic feedback. The control design, for the first time, provides a methodology to integrate US imaging in an FES control framework, which will likely benefit persons with drop foot and those with other mobility disorders.
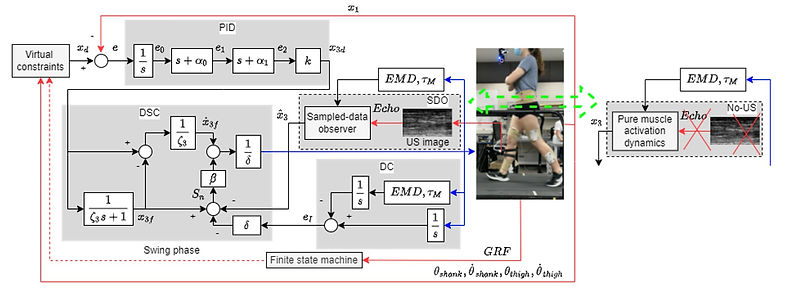
Fused ultrasound imaging and surface electromyography-based control of a cable-driven ankle exoskeleton
Integrated with the above neuromuscular modeling-based ankle joint dynamic net plantarflexion moment prediction, I have developed an assist-as-needed (AAN) control framework for exoskeleton assistance. An adaptive impedance controller (AIC) manipulates the exoskeleton’s impedance for a more natural behavior by following a desired intrinsic impedance model. We have performed treadmill walking experimental studies on five participants with no neurological disabilities to demonstrate the effectiveness of the designed ankle exoskeleton and the proposed AAN approach. Results illustrate that the AAN control approach with fused sEMG and US echogenicity signals maintained a higher human volitional effort prediction accuracy, less ankle joint trajectory tracking error, and less robotic assistance torque than the AAN approach with the sEMG-based volitional effort prediction alone.
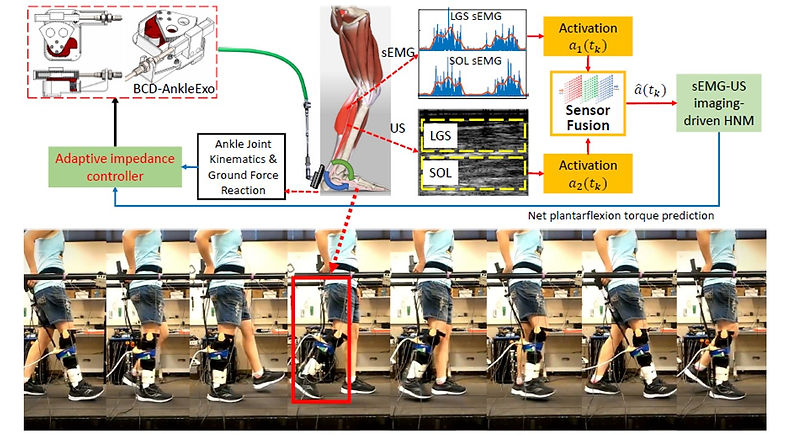
Iterative learning control for a hybrid exoskeleton+FES neurorehabilitation system
I have collaborated with colleagues from the Neuromuscular Control and Robotics Lab to explore a switched distribution of allocation ratios between FES and electric motors in a closed-loop adaptive control design. The new controller uses an iterative learning neural network (NN)-based control law to compensate for structured and unstructured parametric uncertainties in the hybrid exoskeleton+FES dynamic system.

Model predictive control for FES-actuated antagonistic muscles on human elbow joint
I have collaborated with colleagues from the Neuromuscular Control and Robotics Lab to develop a model predictive control approach for human elbow joint trajectory tracking task by using FES on antagonistic muscles, including biceps brachii and triceps brachii muscles. The experimental results on four healthy participants indicate that the controller is robust and effective in switching between antagonistic muscles.

Machine learning-based method for extracting morphological features from B-mode ultrasound images
B-mode ultrasound (US) is often used to noninvasively measure skeletal muscle architecture, which contains human intent information. Extracted features from B-mode images can help improve closed-loop human–robotic interaction control when using rehabilitation/assistive devices. The traditional manual approach to inferring the muscle structural features from US images is laborious, time-consuming, and subjective among different investigators. We propose a clustering-based detection method that can mimic a well-trained human expert in identifying fascicle and aponeurosis and, therefore, compute the pennation angle. The clustering-based architecture assumes that muscle fibers have tubular characteristics. It is robust for low-frequency image streams. We compared the proposed algorithm to two mature benchmark techniques: UltraTrack and ImageJ. The performance of the proposed approach showed higher accuracy in our dataset, that is, similar to the human expert. The proposed method shows promising potential in automatic muscle fascicle orientation detection to facilitate implementations in biomechanics modeling, rehabilitation robot control design, and neuromuscular disease diagnosis with low-frequency data stream.
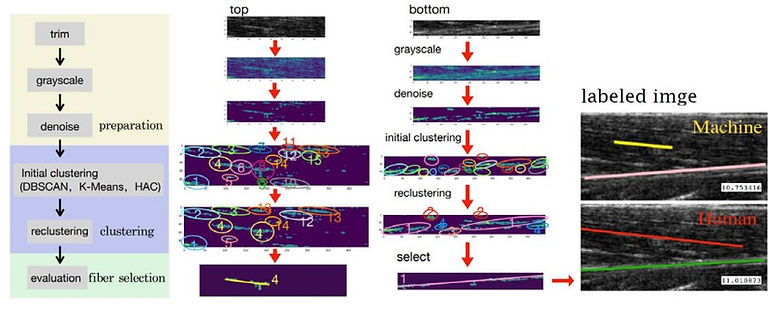
Ultrasound imaging based modeling and muscle fatigue detection during FES
Hybrid neuroprostheses integrate functional electrical stimulation (FES) of leg muscles with electrical motors to generate torque in the lower extremities. Evaluation of FES-induced muscle fatigue is required to properly coordinate these two systems. Traditionally, this fatigue estimate is obtained from fatigue governing equations, which are highly dependent on offline force measurement during isometric contraction experiments. Ultrasound imaging technology potentially provides an alternative online method for fatigue estimation which is a more direct, accurate, and natural way to characterize system behavior in a real-time hybrid neuroprosthetic control system.

Research on bionic mechanism and optimization design methodology of skeletal muscle-like variable stiffness actuator
-
Design of the hardware and control platform of a portable upper limb exoskeleton prototype with 4 active DOFs and 2 passive DOFs, where active DOFs were actuated by series elastic actuators (SEAs) and Bowden cables.
-
Development of a feedback linearization control strategy that is used for SEA motion tracking control and force control, as well as upper limb exoskeleton impedance control.
-
Human-exoskeleton-interaction
-
Assist-as-needed control for upper-limb exoskeletons.
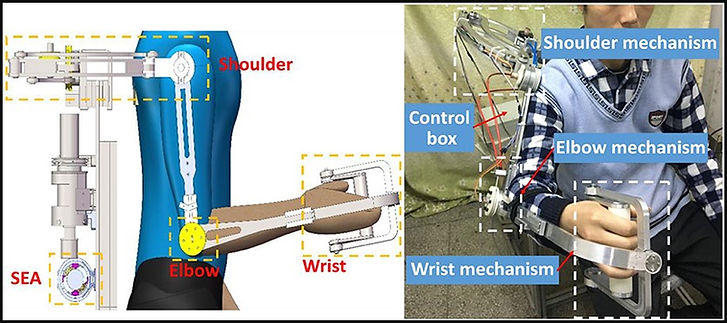
Research on 4-DOFs Underactuated Bipedal Walking Robot
-
Hardware design of an underactuated bipedal walking robot with 4 active DOFs and modeling of the prototype’s equivalent multiple rigid links dynamics for the periodic stable off-line gait planning based on Poincáre return mapping method.
-
Development of the National Instrument single-board RIO-based control system of the bipedal walking robot, and LabVIEW programming to control the robot walking locomotion at a hung state in an open-loop manner.
-
Collaboration on a human-inspired adaptive feedforward control strategy to stabilize the robot’s underactuated bipedal walking locomotion on a compliant ground.
